What impact do surface shadows have on a point cloud generated using photogrammetry?
- Kenny McLean
- Dec 13, 2016
- 5 min read
Purpose
This brief is a high level overview of the potential problem posed by shadows when creating a surface model using photogrammetry. Since photogrammetry is based on still images and therefore can only derive data from what is ‘seen’ by the camera. If high contrast areas exist because of meteorological lighting conditions, can these areas impact our resulting point cloud 3D model? Will this variance introduce error into the elevation model?
This high level overview of the topic is provided solely as introductory guidance for our pilots and planners.
Methods
A suitable target area was chosen that has a uniform surface and contains the requisite shadow. For this we used a groomed pasture with a large high transmission concrete pole. This concrete pole has a base diameter of about 1.5 meters, over 35 meters in height and is tapered.
For this brief an eBee RTK was used and the imaging payload was Canon S110. Flight over subject area was conducted at 1300 hours (GMT -5), which provided high solar irradiance of our surface target and sharp contrast on the landscape. Meteorological conditions provided clear sky and low wind (<8 knots).
All data processed in the WGS 84 datum as it will be satisfactory for our purpose. The mission altitude was flown to achieve ground resolution of 5cm / pixel. Mission overlap was set to 70% lat / 80% long and the target area flown with perpendicular legs.
Initial processing was conducted using Pix4D. Settings that deviate from standard 3D Map template are: Image Scale was set to 1 Original Size, point density High, No default classification of data points.
Orthomosaic
Pictured below (Image 1) is the ortho derived from the mission. This is provided to assist with orienting our readers to the target area. In this image our subject shadow is visible and contrast against the grass.

Image 1
Point Cloud
Our point cloud of the grass area renders well (Image 2), and we see tight registration between the data points. It is difficult to view effectively using a static imagery, but in Image 3 (Low Angle) there appears to be a sizable amount of ‘noise’ in the points located along the shadow. Site verification reveals that the grass height is uniform in this area and the surrounding area. (Note: The data points representing the actual utility pole have been removed.)

Image 2 – Point Cloud High Angle

Image 3 – Point Cloud Low Angle
Cross Section Analysis
Analysis of the area in the shadow and the area beside the shadow will reveal any differential in the registration of point data of the surface. We shall examine the shadow regions by using:
A mensuration line along the shadow region.
Cross sections of the shadow region will be exhibited.
Image 4 illustrates the layout of mensuration lines around our target shadow region. These are ordered left to right with the left being the starting point at 0 meters and to the right at about 16 meters distant from the concrete pole.
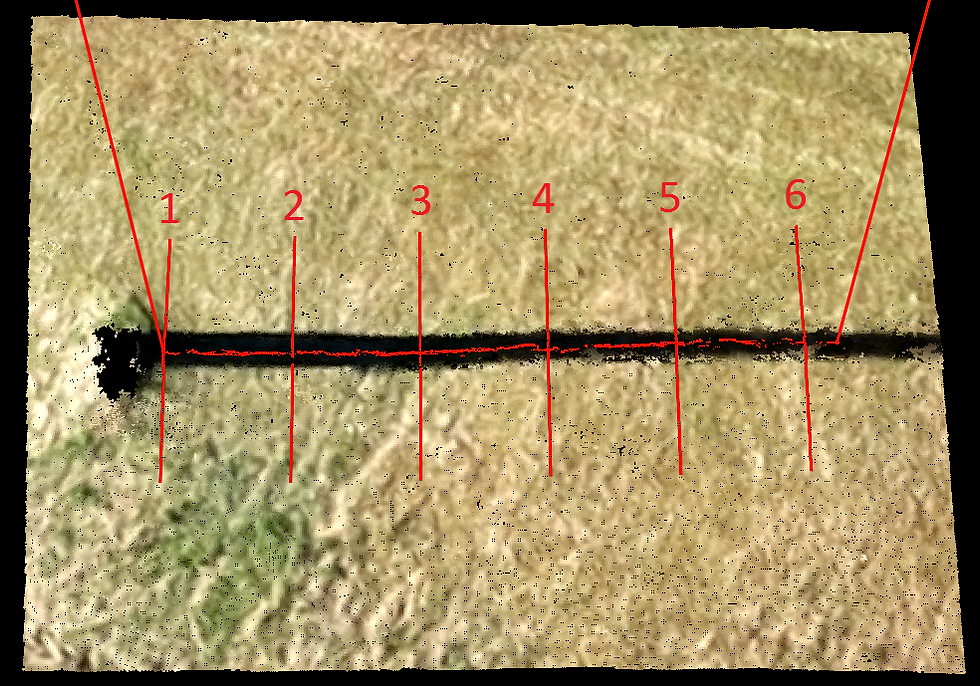
Image 4 – Mensuration line
Image 5 below shows how noisy the point cloud is within the shadow region. The sample of data points represented below (Image 5) is generated by a path that is about 0.5 meter wide along the centerline of the shadow. Image 5 clearly illustrates that as our distance from the pole base increases the less coherent the point data becomes. Image 5 further illustrates, that as we get farther away from the pole base there is less coherence in the resolution of derived point cloud. This is likely induced by a smaller ratio between the umbra and penumbra of the shadow (fuzziness) when processing the images.
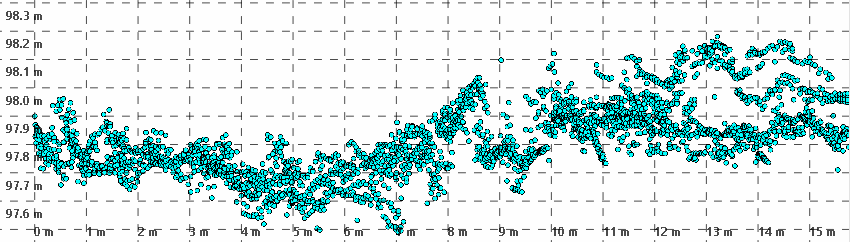
Image 5 – point cloud sample along center of shadow
In Image 5 we can determine that the point cloud data has low correlation within the shadow. How does this relate to the well-lit grassy areas surrounding the shadow region? The images that follow are generated by point data along the shadow cross sections defined in Image 4 (listed as lines 1 – 6). These cross sectional areas are about 6 meters in length, the capture width is 0.15 meters and each is spaced at 3 meter intervals. Each image shows relatively tight point cloud registration until we get around the mid-point (the shadow area) at 3 meters.
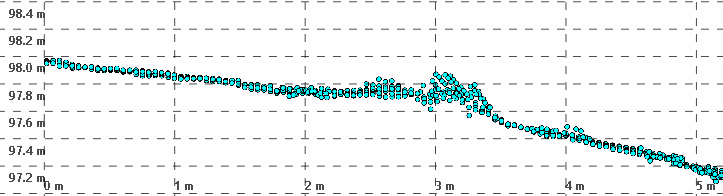
Image 6 – Points from Cross Section 1.

Image 7 - Points from Cross Section 2.
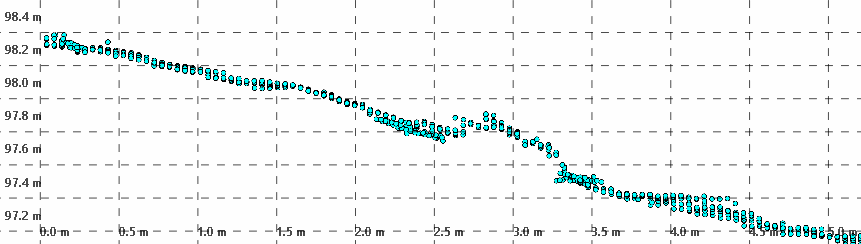
Image 8 - Points from Cross Section 3.
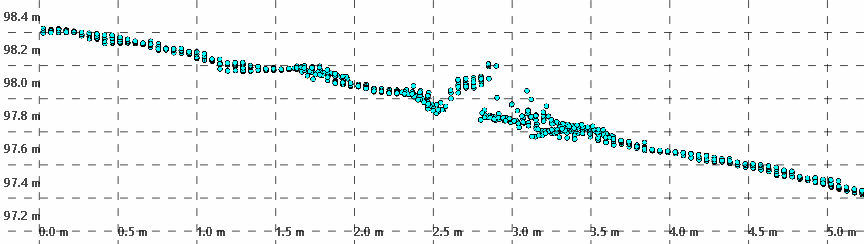
Image 9 - Points from Cross Section 4.

Image 10 - Points from Cross Section 5.
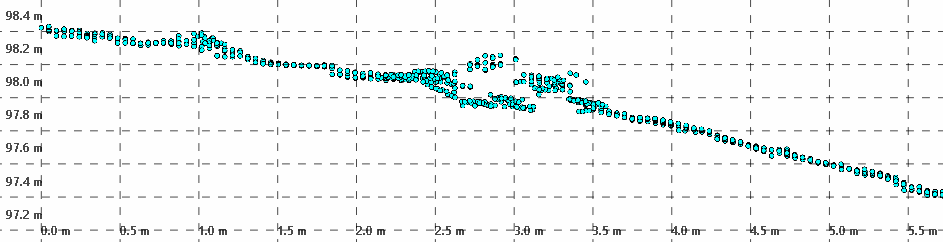
Image 11 - Points from Cross Section 6.
Interpretation
In images 6 through 11 it is clear that the illuminated grass region (areas exclusive of 2.5 meters – 3.5 meters) has tight cohesion in our population of point data. Analyzing the shadow region of 2.5 meters – 3.5 meters, it is clear that point data cohesion loosens. Image 5 (generated from the center line of the shadow) one can see that the point data cohesion is relatively weak along the entire length, clearly worsening as we get farther away from the base.
Takeaway / Conclusion
It is clear that strong shadows can impact the accuracy of the derived point cloud within the shadow region. Since all post processing is based on the accuracy of the point cloud, pilots will need to observe their target area / surfaces and make field accommodations in flight planning to best handle missions with strong shadows that may exist in critical areas.
Delay time between images containing a shadow effect as a result of long flight legs can make the shadow appear to move when processing. Time of day can be used to address the location of shadows if located in a critical or undesirable area. Another option is to adjust camera settings to better handle the contrast.
However, some surfaces or conditions may require LIDAR as the best solution to meet the customer’s needs. Always set customer expectations so that they have an accurate and thorough understanding of what the capabilities are and any limitations that may exist.
Extra Credit: Affects on DEM
Since the point cloud accuracy is proven to suffer in areas such as our example shadow, anything that depends the accuracy of the same cloud will be impacted as well. Since almost everything is derived from the point cloud, care must be taken in post processing to determine what impact these inaccuracies will have. Let’s test the boundaries of the impact by creating two example DEMs from the point cloud used in this sample data set.
The first DEM created by using a small grid size of 0.05 meter and removing any spike over 0.3 meter. Image 12 (viewed from the opposite end of the shadow from the pole) shows that the noisy area within the shadow left a large quantity of false elevation returns along the distant end of the shadow. The end closest to the actual pole is still showing elevated from the grass but not quite as exaggerated.

Image 12 – DEM generated using grid size of 0.05 meter.
The second DEM created (Image 13 below with the same view perspective as Image 12) shows the results of the DEM generated using a larger grid size. This DEM is based on 1.0 meter grid size with spike removal over 0.1 meter. So by increasing the grid size and more aggressive spike removal, we can clearly flatten the data points in the shadow region. This would be considered a ‘low resolution’ DEM for this sample size, but does reduce the impact of the low cohesion created in the shadow region.

Image 13 – DEM generated using grid size of 1 meter
As DEM creation is clearly impacted by the point cloud cohesion, when post processing it is vital that the proper quality control(s) be put into place to ensure that the adequate review of all high contrast areas as dictated by the customer’s need.
Clearly the higher the resolution of the surface required then the more attention must be paid to any high contrast surface present in the project area.